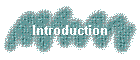



|
|
Requirements
To carry out this project the following were needed:
1. LandSat satellite Imagery of Path 19/31
on the required dates ie July 16, 2002 and August 01, 2002
2. In-situ data for the same dates
3. Image processing software
LandSat imagery was acquired from Ohioview
on the required dates ie., August 1, 2002 (Path 19/31), August 8, 2002(Path
20/31) and July 16, 2002(Path 19/31). The in-situ turbidity data was
acquired from the University of Toledo for the above dates.
ENVI 3.4 was chosen for
image processing as it had some useful functions built into it i.e.
BandMath etc. A methodology (Fig.3) was developed before executing the
project in order to approach the problem in a mannered way.
 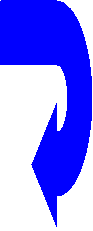  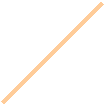   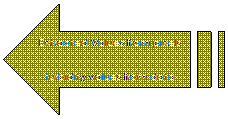 
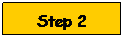 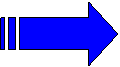  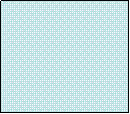  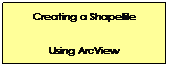 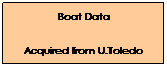 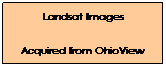 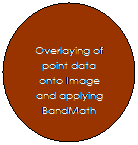 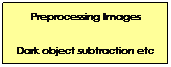

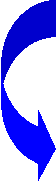
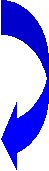
Overlay of boat data and Raster data |
 
Now going through step by step
process........
Step 1
Collection of data:
The required data ie boat data
and cloud free LandSat Imagery were acquired
Step 2
Assumptions:
1. The atmospheric conditions
were assumed to be the same during the boat data collection and satellite
overpass
2.The wind direction and water current conditions
are assumed to be same during the satellite overpass and data collection
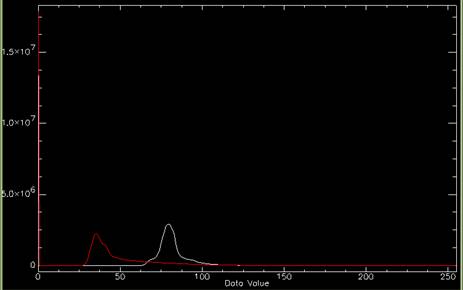
Dark Object Subtraction:
Examine brightness values in
an area of shadow or for a very dark object (such as a large clear lake) and
determine the minimum value. The correction is applied by subtracting the
minimum observed value, determined for each specific band, from all pixel
values in each respective band. Since scattering is wavelength dependent the
minimum values will vary from band to band. This method is based on the
assumption that the reflectance from these features, if the atmosphere is
clear, should be very small, if not zero. A histogram after dark object
subtraction for the same image can be seen in the Fig.5 below. |
 
Fig. 4 showing the digital numbers of
band 3 and 1 of LandSat before Dark Object Subtraction |
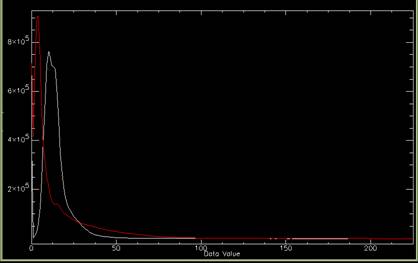
 
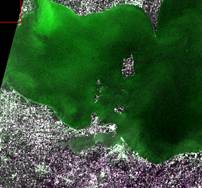
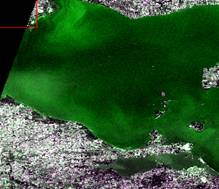
Fig.5 showing the digital numbers of
band 3 and 1 of LandSat after Dark Object Subtraction |
Spatial and spectral subset:
Spatial and spectral subset
were made to the dark object subtracted images. The image is subset
spatially and spectrally to decrease the processing time and memory. It was
found out empirically that Band 3 and Band 1 of LandSat ETM are closely
related with turbidity values (refer Fig.6). Hence the images were
spectrally subset for bands 1 and band 3.The processed images could be seen
in figures 7, 8&9 below. |
Step 3
Preprocessing of the images:
The images acquired were first
preprocessed using some of the basic tools in ENVI 3.5. Dark Object
Subtraction was applied to the images. The atmosphere introduces two forms
of path radiance into the signal, radiance from Rayleigh or molecular
scatter, and radiance from aerosols or haze. These can be removed
simultaneously using dark object subtractions (refer Fig 4).
|
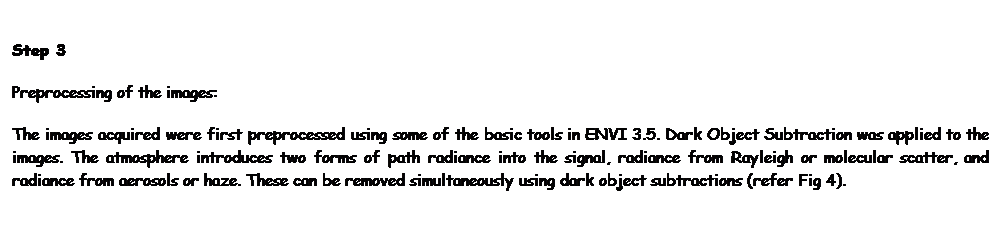
Boat data:
The in-situ boat data was
collected in the western basin of Lake Erie at 28 points on two dates i.e..,
July 16, 2002 and Aug 1, 2002. The data includes the sechhi depth
measurements converted to NTU (Nephelometric Turbidity Unit). The points
have longitude and latitude collected with a GPS unit. The data is added to
ArcView 3.3 to create a point shapefile with the 28 points of in-situ
measurements. The point layer is then projected to the coordinates of the
image i.e.., NAD 1983 datum.
Step 4:
The file is then brought into
ENVI3.5 and was overlaid onto the preprocessed image (refer fig 10 & 11).
|
Fig.6 Reflectance curves of LandSat 7
ETM+ Bands 3 and 1 .Click on the image to see a detailed picture |

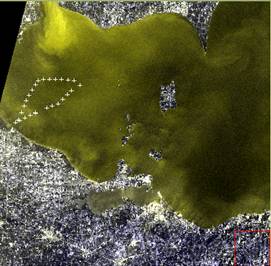
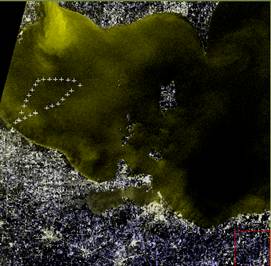
Fig.10 Point data overlayed onto
preprocessed LandSat 7 ETM+ image of July 16, 2002 |
Fig.11 Point data overlayed onto
preprocessed LandSat 7 ETM+ image of August 1, 2002 |
Step 5:
Brightness values were
extracted from the satellite digital data and were used to develop
regression models with in-situ sampling . Linear regression models were
developed from brightness values and onsite sampling data. Data from the 28
individual sampling sites were compared with the average brightness of the
red (band3) and blue (band1) reflectance of water and turbidity. Histograms
and tests of normality indicated the distributions of satellite sensor data
were normal for the study area. a band math function was developed using the
prior empirical functions derived by Czajkowski et al. The in-situ data was
overlaid onto the images after reprojecting the point data into UTM (NAD
1983)and a new empirical model was developed using BandMath function in the
image processing software ENVI 3.5 and was applied to the band ratio 3/1. An
average of 9 pixels i.e. 3x3 matrix from BandMath applied LandSat image
(each pixel is 30X30 mt) was taken as mean value for each of the 28 in-situ
observations. Mean averages for all the 28 observations were taken.
Regression analysis was applied to the data. The mean values with negative
numbers were assumed to be that of clear waters and thus were not considered
when running the analysis. After the analysis the BandMath processed images
are made into turbidity maps reflecting the clarity of water. Fig 12 and Fig
13 represent the two turbidity maps i.e. July 16, 2002 and Aug 01, 2002. The
darker tones represent more turbidity and the lighter tones represent low
turbidity.
|
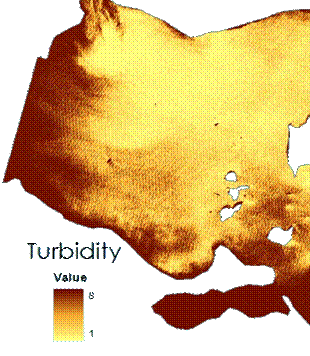
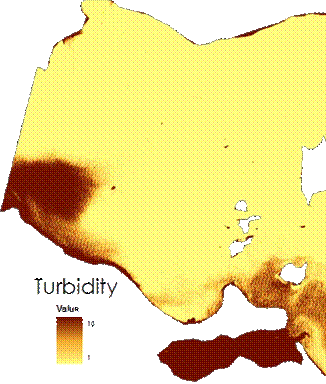
Fig.12 Turbidity map
of July 16, 2002 showing the turbidity. Darker tone represents more turbid
waters
|
Fig.13 Turbidity map
of Aig 01, 2002 showing the turbidity. Darker tone represents more turbid
waters
|
|